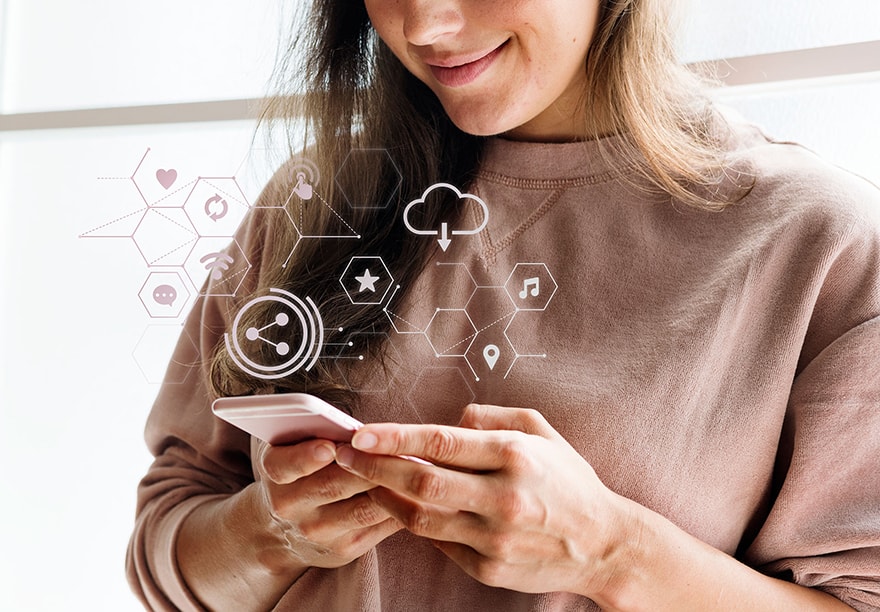
Our panel shared three core steps for getting started with AI. Each step will make AI easier to implement and more effective.
1. Start small
Find practical use cases and pick a pain point to prioritize them. Ensure that whatever use case you pick is small enough to be practical but painful enough that the solution is measurable and impactful for stakeholders.
According to one of our panelists, Kieran Gilmurray, Global Automation and Digital Transformation Expert at Mercer, one advantage of the insurance industry is that use cases are plentiful, proven, and validated. Use cases are already well known, so this isn’t a place where you need to innovate.
Olive recommended starting with AI for email classification. Carney agreed: with 20 million emails received a year, and upwards of five minutes spent on each one, email automation presents a big-impact, low-risk opportunity.
The challenge is that customers are regularly emailing customer support with a huge range of questions that can accumulate into long response times. The opportunity is that with AI, you can shorten those response times and become increasingly efficient where other companies are still slow. For early adopters of AI, this advantage can compound, enabling you to outpace competitors and get so far ahead that they can’t catch up.
With AI-assisted email, you can understand incoming communication and predict the following communications. Which means that customers don’t even need to ask certain questions—you’ve already answered them.
Olive says that by starting smaller, you can attack the value chain a little bit at a time.
2. Align to ROI
Any transformative technology requires investment, but the investment required isn’t always a capital one. Capital is important, but more important is the investment in a culture of innovation. You have to encourage a culture that looks past existing methodologies and practices so that they can adopt a technology as game changing as AI.
Both kinds of investment require you to think carefully about ROI. To align your ROI vision with reality, you’ll want to frame your ROI analysis with process discovery.
Many in the insurance industry have become accustomed to the number of decision points existing processes contain, which makes them forget just how complex many processes have become.
The potential for AI to handle some of these complex processes is immense, but only if you document and review your businesses first––with data, not anecdotes. The goal of this discovery is to determine where you can provide the most value via AI.
UiPath Task Mining and UiPath Process Mining can both help you understand your processes and their bottlenecks. With these process discovery tools, you can prioritize AI use cases based on measurable business outcomes.
From there, you can begin to broaden your understanding of ROI.
Gilmurray warned that though there are near-term AI use cases, wider transformation will require more than the flip of a switch. Implementing AI involves a path of improvements that eventually lead to significant changes in business operations.
Don’t pressure the team to deliver immediate returns, but do understand the direction of the project and where returns will come from. AI is similar to, in a sense, a new employee, meaning it takes time to train it and get it ready for production. And similar to an employee, AI-based solutions can learn and adjust based on the demands of the business.
Olive warned that many companies will overfocus on cost reduction as their primary metric.
The true value of AI, however, isn’t just in what costs it eliminates but what value it produces. AI will, for instance, create better turnaround times and higher customer satisfaction, meaning better customer loyalty.
If you can accurately estimate ROI, from its most narrow returns to its greatest potential value, then you pick the efforts with the most potential every time. You can use a product like UiPath Automation Hub to create a central location for automation ideas that you can then organize and prioritize.

3. Scale by putting business strategy first
For digital transformation to deliver against your enterprise-wide strategic goals, AI adoption can not be siloed within a single team. With the support of both a capital investment as well as a cultural investment, your organization can embrace and extend adoption. If you can’t get widespread adoption, then digital transformation won’t happen, and you risk your AI project stalling as a prototype.
Only a prioritization of business strategy will ensure AI scales and spreads.
The risk is that companies put technology ahead of business strategy. If you focus too much on the technology, Gilmurray warned, then you’ve got the equation wrong. It’s business strategy first.
Gilmurray recommended that you understand the business-level (or at least department-level) strategy to know what you’re contributing to. Technology enables business—it’s people, process and then technology.
You should be asking two questions, he said:
- Where do we want to go?
- How will we get there?
Too many companies focus on the second question without addressing the first.
Insurance is already a data-driven industry, so if you can deliver the right data, at the right time, to the right people, then massive benefits will follow. The ROI calculation won’t only involve the number of hours saved (but that is often times the number-one driver). This calculation will be informed by business strategy and take into account what your competitors are doing and any weak spots in your current business, such as service-level agreements (SLAs) and customer response times.
The U.S. can improve its AI governance strategy by addressing online biases
The United States has been working to codify the National Artificial Intelligence (AI) Initiative that focuses on six strategic pillars: improving AI innovation, advancing trustworthy AI, creating new education and training opportunities through AI, improving existing infrastructure through new technologies, facilitating federal and private sector utilization of AI to improve existing systems, and promoting an international environment that supports further advances in AI. In April 2022, the U.S. Department of Commerce, and the National Institute on Standards (NIST) announced members of the inaugural National Artificial Intelligence Advisory Committee (NAIAC), which will be tasked with advising the Biden administration on how to proceed with national AI governance efforts. At their first meeting on May 4, 2022, the NAIAC discussed the use of AI pertaining to U.S. competitiveness, issues related to workforce, and whether there is adequate national oversight of AI systems. Taken together, the objectives of the national AI initiative and the creation of the NAIAC will ensure strategic and timely approaches to the design and deployment of autonomous systems, as well as further establish national norms.
Of equal importance is that the technology needs to be improved for domestic use cases as part of this national effort, especially in areas with the potential to create either differential treatment or disparate impact for federally protected and other vulnerable populations. If the U.S. excludes such considerations from national governance discussions, historic and systemic inequalities will be perpetuated, limiting the integration of the needs and lived experiences of certain groups into emerging AI innovations. Poor or inadequate decisions around financial services and creditworthiness, hiring, criminal justice, health care, education, and other scenarios that predict social and economic mobilities stifle inclusion and undercut democratic values such as equity and fairness. These and other potential harms must be paired with pragmatic solutions, starting with a comprehensive and universal definition of bias, or the specific harm being addressed. Further, the process must include solutions for legible and enforceable frameworks that bring equity into the design, execution, and auditing of computational models to thwart historical and present-day discrimination and other predatory outcomes.
While the NAIAC is the appropriate next step in gathering input from various stakeholders within the private and public sectors, as well as from universities and civil society stakeholders, representatives from more inclusive and affected groups are also key to developing and executing a more resilient governance approach. In 2021, the Brookings Institution Center for Technology Innovation (CTI) convened a group of stakeholders prior to the NAIAC formation to better understand and discuss the U.S.’s evolving positions on AI. Leaders represented national and local organizations advocating for various historically-disadvantaged and other vulnerable populations.
The goal of the Brookings dialogue was to delve into existing federal efforts to identify areas for more deliberate exchange for civil and equal rights protections. In the end, roundtable experts called for increased attention to be paid to the intended and unintended consequences of AI on more vulnerable populations. Experts also overwhelmingly found that any national governance structure must include analyses of sensitive use cases that are exacerbated when AI systems leverage poor quality data, rush to innovate without consideration of existing civil rights protections, and fail to account for the broader societal implications of inequalities that embolden AI systems to discriminate against or surveil certain populations with greater precision.
In some respects, the roundtable concurred with the need for a “Bill of Rights for an AI-Powered World,” a framework introduced in 2021 by the White House Office of Science and Technology Policy (OSTP). Here, OSTP is calling for the clarification of “the rights and freedoms we expect data-driven technologies to respect” and to establish general safeguards to prevent abuse in the U.S. But without direct discussion on how bias is defined in the public domain, and what specific use cases should be prioritized, the U.S. will wane in the protection and inclusion of historically disadvantaged groups as AI systems evolve.
In this blog, we offer a brief overview of key points from the roundtable discussion, and further clarify definitions of bias that were shared during the roundtable. We also surface scenarios where the U.S. can effectuate change, including in the fields of law enforcement, hiring, financial services, and more. We conclude with priorities that could be undertaken by the newly established advisory committee, and the federal government writ large, to make progress on inclusive, responsible, and trustworthy AI systems for more vulnerable groups and their communities.